Generative AI is revolutionizing the landscape of software interactions. With its ability to create personalized experiences, this technology not only enhances digital interfaces but also completely redefines user expectations. By eliminating traditional technical barriers, Generative AI offers solutions tailored to the specific needs of each individual, representing a monumental shift in software design and making interfaces more intuitive and dynamic.
A new era of human-AI collaboration
Generative AI introduces an entirely new paradigm in software interaction. Instead of adapting to static and rigid systems, users experience applications that continuously evolve based on their needs, thanks to real-time analysis and the generation of personalized responses. Software is no longer just a tool but becomes an active collaborator that adapts to the user.
Imagine a future without complex menus or static interfaces to navigate. Users can obtain customized solutions that respond to immediate needs through simple voice or text commands. Human-AI interaction thus becomes a fluid collaboration, where the user is a co-creator of the technological experience. This evolution not only reduces the time required to perform tasks but also makes software accessible to those without advanced technical knowledge.
The potential of Generative AI
Generative AI goes beyond improving productivity, opening new horizons in creating personalized content and managing complex business processes. Tools like ChatGPT, Midjourney, and Runway - capable of generating text, images, videos, or code - represent just the tip of the iceberg of possibilities offered by this technology. These systems demonstrate how AI can operate as a creative force rather than merely executing predefined instructions.
A fundamental aspect is the ability of Generative AI to learn and improve over time, adapting to user preferences. A virtual assistant, for example, doesn't just respond to specific commands but can anticipate future needs, offering suggestions or automating recurring activities. This predictive interaction significantly enhances efficiency, as the AI can anticipate the user's needs, providing solutions even before they are requested.
Overcoming the limitations of traditional software through Generative AI
Historically, software has imposed predefined and rigid pathways, limiting the user's freedom of action. With Generative AI, this limitation is overcome. Interfaces become dynamic and adaptive, molding themselves not only to preferences but also to the context of use and specific user habits, creating frictionless interaction. This progress leads to a more inclusive user experience, where even less experienced users can easily access complex tools.
Consider the example of an AI agent that proposes solutions and actions based on previously collected user information instead of waiting for specific input. This case isn't just about advanced functionality but about building an interaction that evolves and improves over time, increasing satisfaction and efficiency.
The importance of data integration
Generative AI reaches its maximum potential when integrated into an interconnected digital ecosystem. The power of AI exponentially increases when it can access data from various sources and systems. An AI system can, for example, connect disparate databases to provide personalized solutions, uniting previously separate information. This ability to merge heterogeneous data offers companies the opportunity to create holistic experiences where every customer interaction is informed and optimized.
However, the quality of integration depends on the quality of the data itself. The principle of “garbage in, garbage out” remains fundamental: if the data isn't accurate, the generated solutions will also be incorrect. That's why data management and governance become crucial, requiring special care in collecting, verifying, and using information.
Data governance and ethical responsibility
With the rise of Generative AI applications, new ethical and regulatory issues related to data management emerge. AI algorithms are powerful but entirely dependent on the data they're trained on. If this data contains biases or errors, the generated results will be equally flawed, with potentially serious consequences. Companies need to establish strict governance policies, protecting user privacy and ensuring that the AI produces accurate and contextualized responses.
Compliance with regulations like the GDPR is fundamental, especially when dealing with sensitive data. In a world where Generative AI becomes an increasingly integral part of business operations, companies must ensure that technological innovation goes hand in hand with ethical responsibility.
The role of partnerships in implementing Generative AI
Implementing Generative AI cannot happen in isolation. The most innovative companies are forming strategic partnerships to maximize AI's potential. E-commerce platforms, for example, are already using specialized AI agents that enhance customer interaction by providing product recommendations based on individual preferences, creating a more personalized customer experience.
In this context, collaboration between companies, technology providers, and commercial partners becomes crucial to creating an interconnected ecosystem. This integrated approach not only improves operational efficiency but also offers added value to the customer by providing tailor-made solutions in the context of continuous improvement.
Choosing the right Generative AI project
With the proliferation of large language models, companies face significant opportunities but also important decisions on how best to implement these technologies. To avoid risks and maximize benefits, it's essential to evaluate both market demand and potential risks carefully.
Step 1 - Assess demand and risk
A practical approach involves using a demand-risk matrix to classify projects based on their potential for success.
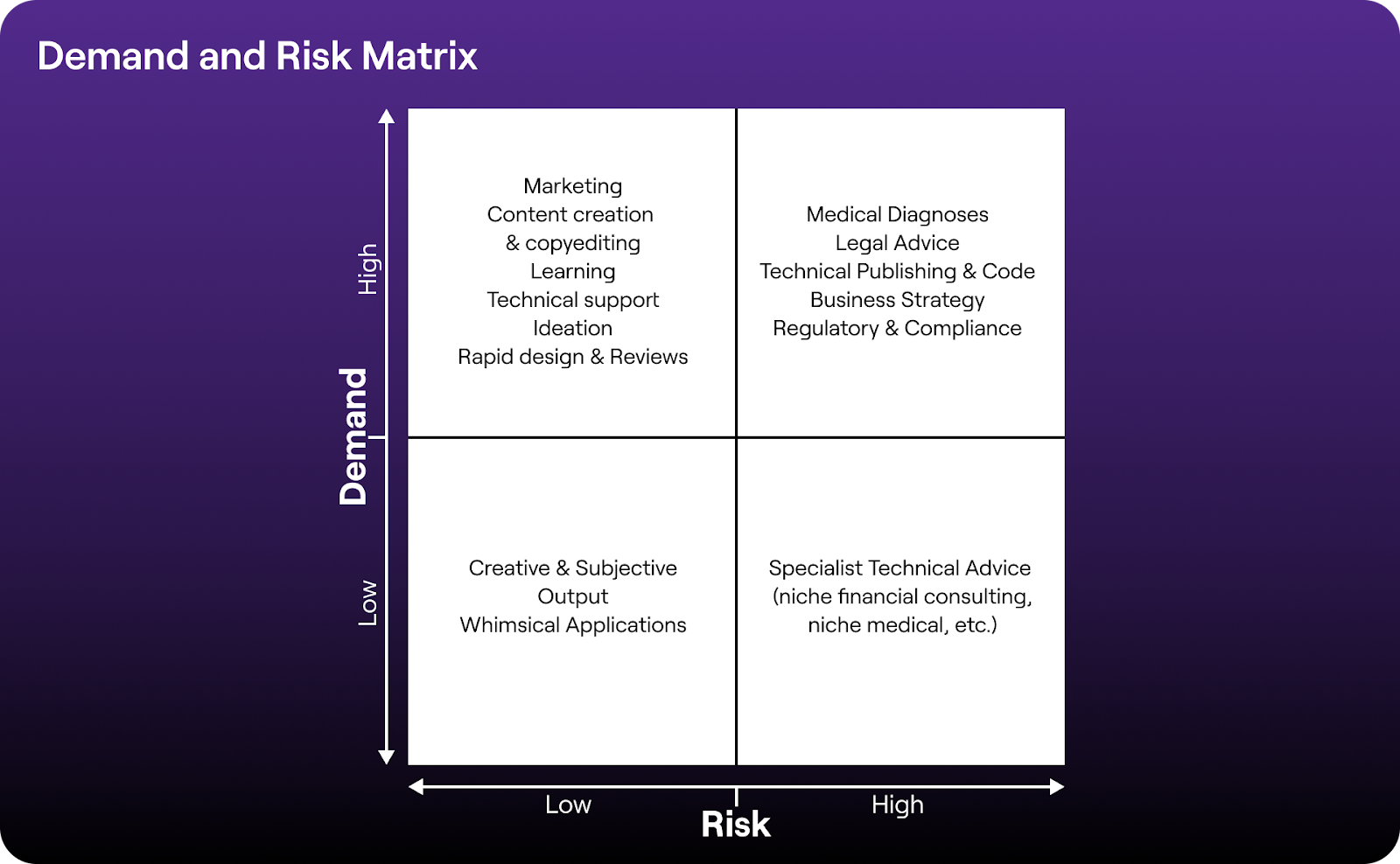
- High demand and low risk
Projects that can be implemented quickly and without much difficulty, such as creating marketing content. - High demand and high risk
These projects require continuous human validation, like training texts or legal documents generated by AI. - Low demand and low risk
Easily implementable solutions with limited impact, such as managing FAQs through AI. - Low demand and high risk
Projects to avoid, like using AI for critical strategic decisions without adequate human oversight.
Step 2 - Identify use cases
Once you understand the project's position in the demand-risk matrix, the next step is to identify the most appropriate use cases for your company. These may include content creation, staff training and learning, or customer service and sales through AI agents.
Step 3 - Implement human validation
Even in low-risk applications, it's essential to incorporate a phase of human validation. AI-generated outputs must be carefully reviewed to correct any errors or biases. For example, in marketing, AI can create creative proposals, but human teams must supervise the content to ensure consistency with the brand.
Step 4 - Continuous improvement
Adopting Generative AI is not a "plug-and-play" process. The initial output provided by AI can be a good starting point but requires iteration to optimize results. Companies must be ready to refine the prompts and instructions given to the AI, test different use cases, and collect feedback to identify weaknesses and opportunities for improvement. Additionally, integrating human intelligence in the final iteration ensures that outputs are creative and contextualized.
Step 5 - Monitor results
Once Generative AI is implemented, monitoring Key Performance Indicators (KPIs) is crucial to ensure the project produces the expected results. Some useful KPIs to monitor include user satisfaction rate, average time to handle requests, and automation rate. We've discussed this more thoroughly in this article.
Generative AI is not just the future of software; it will also redefine the future of business. Companies capable of fully harnessing this technology's potential will optimize their operations and emerge as leaders in the new digital landscape. We are witnessing the beginning of a new era of dialogue between brands and customers, where authentic conversations lead to deep and lasting relationships.
FAQs
What are the main advantages of integrating Generative AI into software?
Integrating Generative AI into software offers numerous benefits. It personalizes applications by adapting them to individual preferences, increasing user satisfaction and engagement. It enhances operational efficiency by automating repetitive tasks and predicting future needs, reducing time and resource expenditure. Generative AI fosters creativity and innovation by generating new ideas and solutions within the organization. Additionally, it improves accessibility by making software more intuitive and user-friendly, even for those without advanced technical skills, through natural and interactive interfaces.
How can a company start implementing Generative AI in its processes?
To begin implementing Generative AI, a company should first assess demand and risk by identifying projects with high potential impact and manageable risk. Next, it should identify use cases where AI adds the most value, such as marketing, customer service, or internal training. Implementing human validation is crucial to ensure that humans review AI outputs to correct errors or biases. The company should commit to continuous improvement by refining AI prompts and instructions, testing different use cases, and gathering feedback. Finally, it should monitor Key Performance Indicators (KPIs) to evaluate effectiveness and make necessary adjustments.
What are the main ethical and data governance challenges related to Generative AI?
The main challenges include data bias, which poses the risk of perpetuating prejudices if AI is trained on non-representative datasets. Privacy and data protection are critical, necessitating compliance with regulations like GDPR due to the large amounts of data required. Transparency is a concern because of the lack of explainability in AI decisions, requiring transparent processes. Accountability involves determining responsibility for AI actions, especially in cases of error. Security is essential to protect AI systems from manipulation or attacks to maintain their integrity.